Let us stop the hypocrisy of nicely matching experimental validations
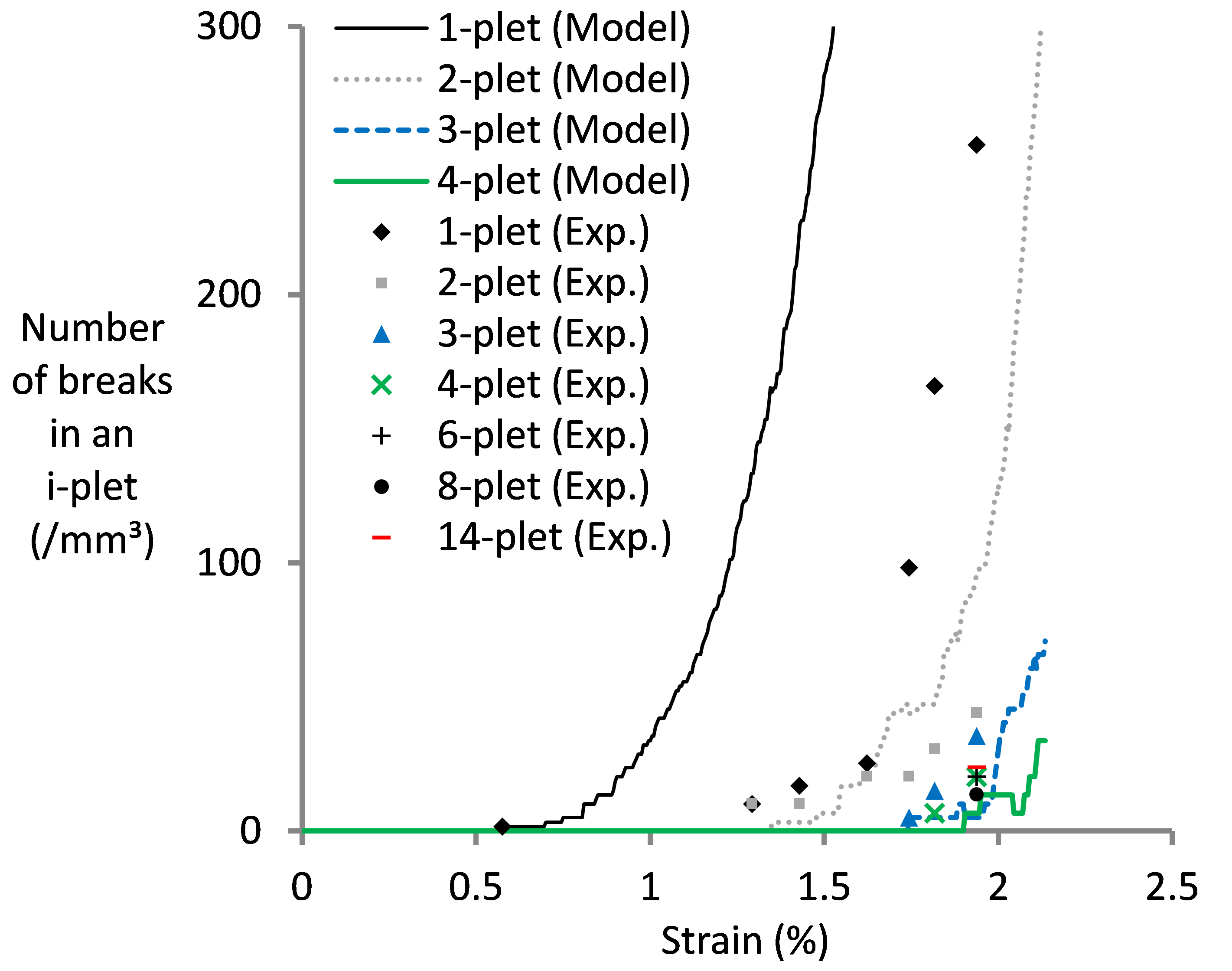
Let us stop the hypocrisy of nicely matching experimental validations
Experimental validations are vital to any model development. This is also why it ended up to be a key component of the FiBreMoD project. For a long time, I have felt that models for composite failure have been insufficiently validated by experiments. I believe this has several reasons:
- Models are typically validated by simply looking at failure predictions, and rarely by comparing the internal damage development leading to failure.
- Gathering input data for such models is particularly difficult, and often not done properly.
- Failure of composites often involves interacting mechanisms, leading to models that require many input parameters.
Point 1 is extensively addressed within FiBreMoD. Our project tries to exploit recent developments in synchrotron computed tomography to address this issue. Some of our past work (see image below from Swolfs et al. Composites Part A 77 (2015) p. 106-113) has already revealed new insights into how composites fail. More importantly, that work showed that despite predicting the final failure reasonably well, the internal damage development was severely off. This is a vital conclusion, as it severely hampers the predictive capacity of models. The true power of models lies in their predictive capacity based on independently measured input parameters. How can you be sure that other predictions are accurate if you do not predict internal damage development properly? In the end, we learn much more from predictions that do not match than from predictions that do match.
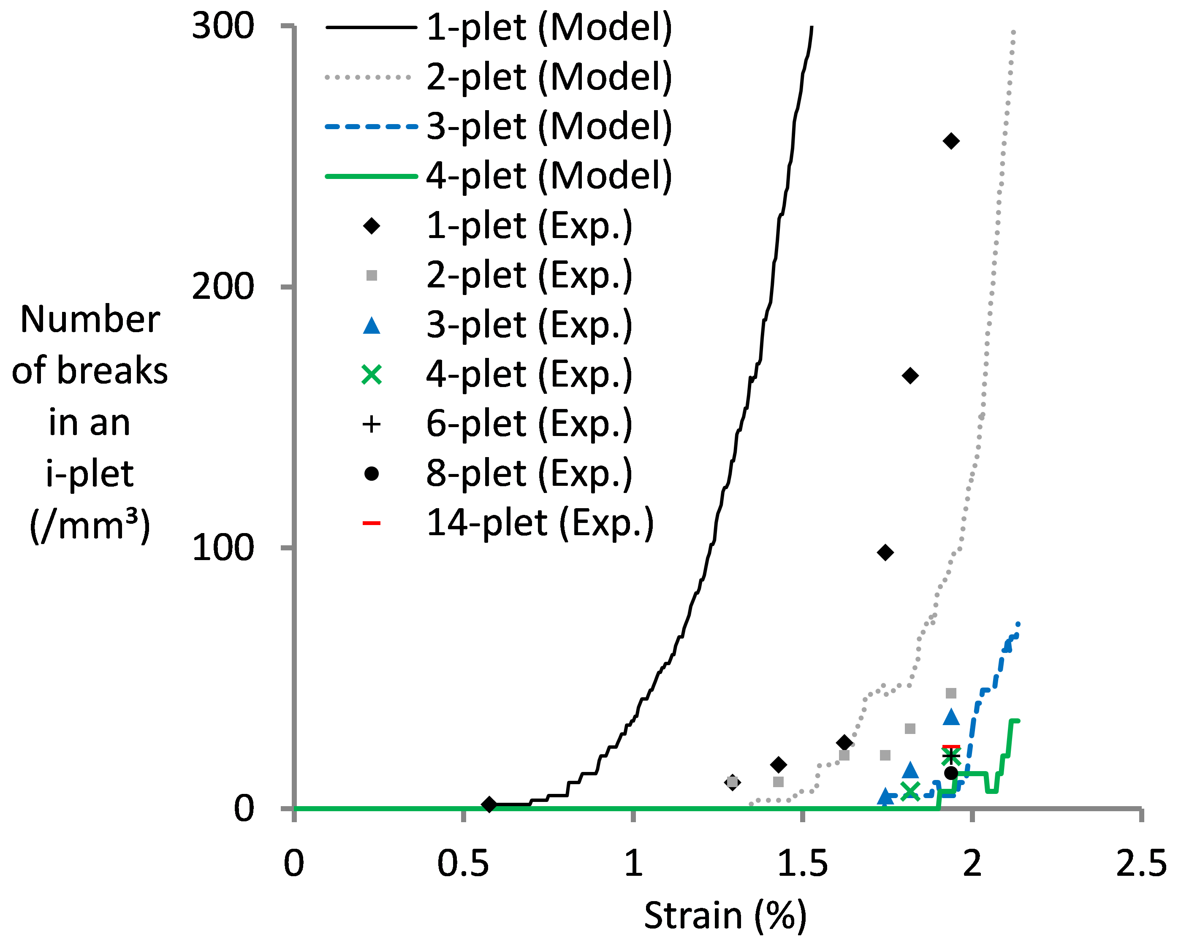
Points 2 and 3 are the real reasons why I am writing this blog post. I recently read several papers that claim an excellent match between model predictions and experiments. Such papers are easy to publish, as reviewers and editors tend to like them. That is probably also why they seem to be appearing more commonly in recent years, and why some people or groups seem to be specialise in them. However, I feel that they convey the wrong message to the composites community. Knowing how many input parameters they require and how difficult it is to reliably measure those input parameters, getting a good agreement is at best a matter of luck. At its worst however, it is a matter of (1) intelligently choosing which input data to take from the literature, (2) cherry-picking literature data that you compare your predictions with, and (3) implementing additional features to models until the predictions fit with the experimental data. I completely realise this seems like a grim perspective on both the honesty of authors and the accuracy of modelling predictions. There is definitely also an aspect of human psychology playing here: the confirmation bias. Humans have a tendency to look for information that confirms their existing views and to ignore information that rejects those views. The pressure to publish and to improve your academic track record also plays a key role. If your experiments do not match your models, then it is much more challenging to publish. We have experienced this ourselves, and also heard similar stories from other researchers in the field. This is recently getting worse due to the increased push to combine experiments and models in paper.
What can we do about this? I wish I had a clear-cut answer, but regrettably, I do not. Nevertheless, I can make some suggestions:
- Authors should more extensively describe the details of their model to improve reproducibility. Very often, not all parameters are mentioned, which hampers reproducibility and accountability.
- Authors tend to hide or forget the limitations of their experimental validations, as this makes it more difficult to get past reviewers and editors. I am therefore calling upon authors to be more open about this and on reviewers and editors to more critically assess this aspect of manuscripts.
- I suggest that in experimental validation papers, authors use different input parameters from the literature and show how this affects the results. This helps to avoid cherry-picking of input parameters until the predictions match the results.
- Reviewers and editors should be more open to accept papers which do not offer a good match between experiments and predictions. They often teach us much more than papers where the match is excellent.
- The evaluation system in academia should shift from quantity to quality.
- Leaders in the field should lead by example. We certainly intend to do this ourselves, and to keep emphasising this issue.
This blogpost represents my personal opinion. I hope that it creates a starting point for more detailed discussions and for some authors to revise their approach to research. I look forward to hearing your own opinion, please do get in touch with me.